Innovating the new Globetrekker report took months of time and careful curation of a huge phylogeographic dataset. Once the method was invented, the FamilyTreeDNA R&D Team located tens of thousands of haplogroups through time to determine each one’s migration path.
This is part two in a three-part series about Globetrekker, phylogenetics and human history. Read part one here:
Hidden in our collective DNA is the story of humanity’s trek across the globe. Big Y customers are revealing the legacy of a massive family tree stretching back to the dawn of humanity in Africa. Our shared ancestors journeyed across the African savanna, entered the gates of the arctic, and paddled through the Pacific.
Globetrekker, our new tool, offers a giant leap forward for the science of phylogeography. Coined in 1987, phylogeography aims to map the spread of ancestral lineages across the planet. Our goal is to infer (pre-)historical migrations using a combination of present-day locations, and ancient samples. Short of inventing a DNA-swabbing time machine, we must use sophisticated methods to infer the locations of our shared ancestors.
Note: modern statistical phylogeography usually considers genome-wide (population) spread, whereas Big Y customers are solely exploring their patrilineal history.
Our newest Discover™ method is unique by considering:
- The largest available dataset
- Ancient DNA
- Sampling bias
- Past sea levels
- Terrain
- Coastal routes
- Sea currents
- Glacial boundaries
- Least cost migration paths
- Corridor methods published in Heredity
Step 1: Modeling the Past Environment that Influenced Human Migration
There are many initial steps to filter and curate our customers’ geographic data, and publicly available environmental data.
First, we must know the extent of continental land masses through time. Global sea level was lower during the last ice age, creating land bridges such as Beringia, Doggerland, Sundaland, and the Sahul continent.
We can apply past sea levels to a global bathymetry layer to reconstruct the shape of the continents at any particular time period. If the entire globe is like a bathtub, then bathymetry tells us the shape of that bathtub. Our global bathtub is filled with contoured terrain (continents) poking up through the waterline. The waterline (sea level) has fluctuated between 0 and -139 m (24,000 years ago). So bathymetry allows us to know which land bridges existed and when. Shallow seas today were fertile ground for migration and settlement thousands of years ago.
A similar process is applied to glacial boundaries, which also provided or inhibited migration corridors such as the Amerindian expansion.
Not all human migration occurred entirely overland. Some cultural movements, such as the Viking raids, and the Austronesian expansion, used seafaring routes. When such routes were mostly coastal, we assume they followed as closely to existing land as possible.
“Coastal” routes (those within 200 km of land) are thus simulated to prefer a path that minimizes offshore distance. However, oceanic routes that are farther seaward may be subject to maritime conditions. We use a special layer of oceanic current speeds and directions to simulate the most likely path of least resistance. Afterward, we manually curate these paths and decide whether to keep the path based on sea current or instead display a straight path across the sea.
Step 2: Sample and Tree Filtering to Improve Accuracy of Globetrekker Migrations
Our Big Y Haplotree is a shared collaboration, meaning anyone’s data can potentially affect everyone. Globetrekker is extremely data-driven. This is a strength because it forces us to ignore our preconceived assumptions. However, it also means we must carefully filter the data.
We perform several sample filtering steps.
First, we filter out samples with country/haplogroup combinations that don’t make sense for Pre-Columbian travel. For example, Eurasian haplogroup R1b should not be in the United States, nor should Native American haplogroup Q-M3 be in Europe.
Next, we conservatively filter out “Earliest Known Ancestor” (EKA) coordinates that differ from the listed EKA country. Customers may accidentally provide conflicting geographic information which is a convenient sanity check for us. We do relax this requirement by allowing a 500 km buffer between the two locations. We are also lenient in cases such as the example above: if an EKA coordinate is in America with haplogroup R1b, but the listed country is in Europe, we simply use the country instead. Now is a great opportunity to check your country and coordinate information to make sure it is consistent and correct!
Finally, we check each branch for continental outliers. For example, if a branch contains four samples, with three in Europe but the fourth in South Africa, we rely on the first three.
After samples are filtered, we also filter the tree. We want to carefully ensure that single branches don’t unduly influence the migration paths. If any branch contains only a single geographically informative sample, or its samples are no closer than 2,000 km apart, we collapse that branch.
Step 3: Locating Haplogroups on the Globetrekker Map
Now that we’ve sorted out sample locations, tree structure, and global barriers to travel, we begin the monumental task of drawing ~50,000 lineages accurately onto a map.
Our first goal is to pinpoint the locations of our shared ancestors.
A handful (~100) of the oldest tree branches are anchored using anthropological information from internal and published research. The other ~50,000 branches are automatically placed.
Since we are only interested in Pre-Columbian locations (for now), we utilize the EKA coordinates input by customers in their account settings. (Approximately 2,000 ancient DNA samples are also included.) We assume that each “leaf” of the tree (representing one customer) is anchored by their EKA coordinate. (In the future, we may fine-tune this by associating the EKA birth date with the nearest-dated tree node.) This means that every upstream haplogroup location is unknown.
Using Immediate Downstream Branches For More Accurate Haplogroup Locations
Haplogroups are assumed to be centrally located between their downstream branches. All things equal, people should spread out at similar speeds. However, it’s essential to only consider direct downstream branches to avoid sampling bias.
Consider the example below. The majority of samples (60%) are from the British Isles, so we might naively expect the entire haplogroup to originate there. However, 75% of the immediate downstream branches are Scandinavian. Taking the tree structure into account is important to avoid sampling biases, caused by both family size differences, and database size imbalance.
How do we determine the center (“centroid”) of points?
We find that a weighted median is the fairest approach. Imagine three points in Spain and one point in Ukraine. A median respects the majority rule in this case (Spain), whereas a mean would place the point somewhere in northern Italy.
Weights are also important.
Downstream stems (branches or samples) may have different lengths. Shorter stems imply a shorter amount of time has passed, hence those downstream locations are more informative about their source. For this reason, we upweight shorter stems. For example, an ancient DNA sample from archaeological remains with a known location, and radiocarbon dates close in time to a haplogroup, would have high weight.
Other weights only apply to certain types of stems. For haplogroups containing only samples and no subclades (leaf nodes) we add a country frequency weight to ensure that massively undersampled countries can compete with better sampled ones.
For all other haplogroups, we instead add an uncertainty weight. This helps to downweight the influence of branches with points spread widely across the map.
What happens if the centroid is now floating in water?
We must snap it back to land using some reasonable criteria. Considering the sea level, continent boundaries, and ice sheets at that time, we find contiguous chunks of land nearby. We can safely ignore land chunks containing a tiny share (0–3%) of downstream samples. Then we move the centroid to the closest remaining land chunk.
In summary, a “bottom-up” approach
Thus, we start at the leaves of the tree and work upward toward the root, placing every haplogroup at its centroid, and stopping once we reach an anthropologically curated anchor point. Traversing the tree from leaves to root is often termed “bottom-up”. Once finished, there are two further steps that help improve the previous steps.
The first improvement is called “top-down” smoothing.
Consider the example below, showing haplogroup R-BY342 (purple), its parent R-ZP18 (blue), and its two descendants R-BY336 and R-FT180748 (red). Although this haplogroup and its ancestors have a long English history, one of two descendants is subsequently found in East Germany. That German coordinate would drag it eastward across the channel to the Netherlands. However, we can use its English history as prior information about its likely location. By comparing the distance from grandparent (blue) to grandchildren (red), we can downweight the influence of outliers such as R-FT180748 and place R-BY342 firmly on English soil. This is a more conservative assumption, and smooths out migrational zigzags that would otherwise occur.
The second improvement is called TMRCA spacing.
There is a natural bias toward our method estimating less and less human movement as we approach modernity. This is clearly wrong. The cause is simple: almost none of our samples are more than a few generations old! So by finding averages of averages of sample locations, we might falsely assume that ancient ancestors lived nearby modern people. To combat that bias, we space out the initial haplogroup coordinates according to their genetically determined age (TMRCA, or “Time to Most Recent Common Ancestor”).
TMRCA spacing ensures that haplogroups are spaced out by time.
A path is drawn up the tree, beginning with one leaf node and connecting through each ancestral haplogroup, stopping at the nearest ancestor that is anchored (e.g., I-Z60). Thus, several haplogroups with flexibility are sandwiched between two anchor points. We then space out those haplogroup coordinates according to their time intervals.
Not all haplogroups should be spaced out
Some of those haplogroups also have samples assigned to them that do not belong to any of the subclades (“star samples”). These samples can help guide our certainty about the initial haplogroup location: samples close in time to their haplogroup (e.g., ancient DNA) should carry high weight and prevent much TMRCA spacing from occurring.
Once TMRCA spacing is complete, we have a spaced-out path for each leaf node in the tree. That means each ancestral haplogroup now has at least two (possibly hundreds of) independent coordinates. The final step is to average these together using a “Mean Path Intersect” (MPI). This MPI represents our final estimate of the haplogroup’s location, unless it now resides over water, in which case we snap it back to land. The spatial uncertainty about each haplogroup via the MPI is called a “hotspot” and shown to users.
Step 4: Tracing the Globetrekker Migrations Using Least Cost Paths and Corridors
Did our ancestors all move like Ötzi the Iceman, scaling icy cliffs to reach their destinations via perfectly straight paths? Probably not. We think ancestral humans generally chose routes that avoided terrain. This fact inspired the final innovations of our method: Least Cost Paths (LCPs), and Least Cost Corridors (LCCs). Now that haplogroups are pinpointed, these are the migrational lines connecting them.
Least Cost Paths
LCPs attempt to find the “easiest” or least costly route between two points. Currently our simulations consider three environmental attributes to be costly:
- Steepness of slope (land)
- Distance to land (coastal waters)
- Fast ocean currents, particularly moving in the opposite direction (open ocean waters)
Open ocean water is weighted to be much more costly than land or coastal waters. Nearly all paths will avoid seafaring routes. Those that are naturally seafaring (e.g., Polynesian settlement) might later be manually curated to appear as straight lines, if the LCPs conflict with known historical routes.
Two caveats are worth mentioning. “Land” refers to whatever land was exposed at the time of a specific haplogroup, when sea levels were potentially lower, and some land was covered with ice. Also, over time we may adapt these LCP costs to reflect the best science of the day.
Least Cost Corridors Provide Confidence For Paths
LCCs are akin to confidence levels for LCPs. Our method is inspired by a corridor method recently published in the journal Heredity for the purpose of landscape genetics. The three tiered corridors show the area that is 95%, 96.6%, and 98.3% likely to contain the true path. This of course assumes that slope, ocean currents, and distance to land entirely capture human motivation, which is a simplification. LCPs and LCCs vastly improve upon previous phylogeography by uniquely combining it with this other field.
Globetrekker: The Latest Advancement in Y-DNA Research
Although we anticipate improving our method over time, as new ideas and samples emerge, we are quite proud of this holistic new approach! We build upon our own Tree of Mankind (the largest such phylogenetic tree), our age estimates, Time Tree, Group Time Tree, our growing database of customer research, ancient DNA contributions via Discover™, and previous landscape genetic research.
How Can I Help Improve Globetrekker Migrations?
Remember to check and double-check your EKA coordinate and country, found under account settings. This unprecedented map of human movement relies on the accuracy and consistency of user data.
Stay tuned for the last installment of our three-part Globetrekker series, where we discuss the anthropology behind the tool, and the knowledge gained!
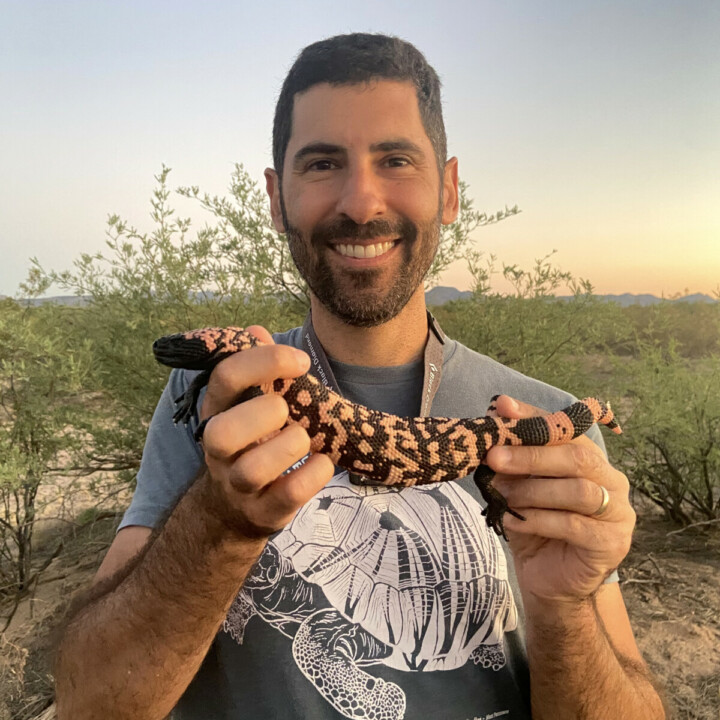
About the Author
Paul Maier, Ph.D.
Population Geneticist for FamilyTreeDNA, Gene by Gene
Dr. Paul Maier is the lead Population Geneticist at FamilyTreeDNA and Gene by Gene, where he builds ancestry estimation tools, and studies the genetic history of human life on earth. Since 2018, he has developed numerous products and features, including myOrigins® 3.0, the Chromosome Painter, Big Y Age Estimates, the FTDNATiP™ Report, the upcoming Mitotree, Geo-Genetic Triangulation (for Beethoven research), and now Globetrekker.
Paul earned his Ph.D. in evolutionary biology, studying the genetic past, present, and future of a much squishier creature, the Yosemite toad in the Sierra Nevada of California. His research used conservation genomics to inform the US Fish & Wildlife Service’s strategy for this federally threatened species. His work is published in journals such as Heredity, Evolution, Frontiers, Evolutionary Applications, Current Biology, and Nature Scientific Reports. While earning his doctorate, he worked as lead biologist for the US Geological Survey, and taught university students about genetics, evolution, zoology, and herpetology.
His scientific outreach tries to emphasize the simplicity of DNA, amidst a complex field. He has given numerous talks, including at RootsTech, Jefferson Public Radio, the Int’l Conference on Genetic Genealogy, Portland ISOGG, and East Coast Genetic Genealogy Conference. He is passionate about using DNA to reconstruct the hidden stories of human and wildlife populations.
Well thought through and clear. Many thanks. One comment on future refinements of ‘Low-Cost’ Migration routes. Early people’s didn’t always migrate using valley routes and avoiding uplands. There are well documented instances of pastoralists avoiding heavily forested lowlands and establishing droving routes using lightly wooded uplands.